In the ever-evolving world of retail, supply chain management has become increasingly complex, with customer demands, market trends, and external disruptions constantly reshaping the landscape.
For businesses aiming to gain a competitive edge, optimizing inventory levels and accurately forecasting demand are critical challenges that must be addressed.
This is where artificial intelligence (AI), and more specifically its branch known as machine learning (ML), comes into play, revolutionizing the way supply chains operate.
The Rise of AI and ML in Supply Chain Management
Over the past decade, AI and ML have transitioned from buzzwords to essential tools in supply chain operations.
With large volumes of data coming from sales, suppliers, and external factors such as weather conditions or geopolitical events, it is now possible to leverage more complex models.
The integration of ML into supply chain operations has recently revolutionized several areas, including inventory optimization and demand forecasting.
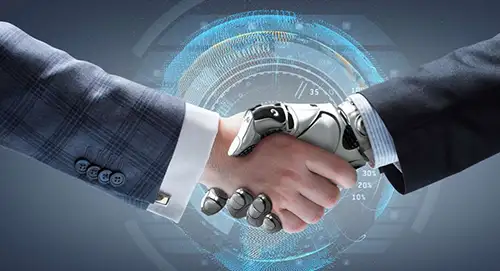
ML FOR DEMAND FORECASTING: A DEEPER DIVE
Demand forecasting is a cornerstone of retail planning, enabling businesses to anticipate customer needs, manage inventory, allocate resources, and develop marketing strategies.
However, accurately forecasting demand remains a notoriously complex task due to the variability and unpredictability of consumer behavior.
Traditional methods, which rely on historical data and basic statistical models, are often insufficient as they fail to account for the multitude of external factors that influence demand.
Machine learning (ML) is reshaping the demand forecasting landscape by providing more accurate, adaptable, and real-time solutions.
Let’s explore how AI is revolutionizing this critical retail function.
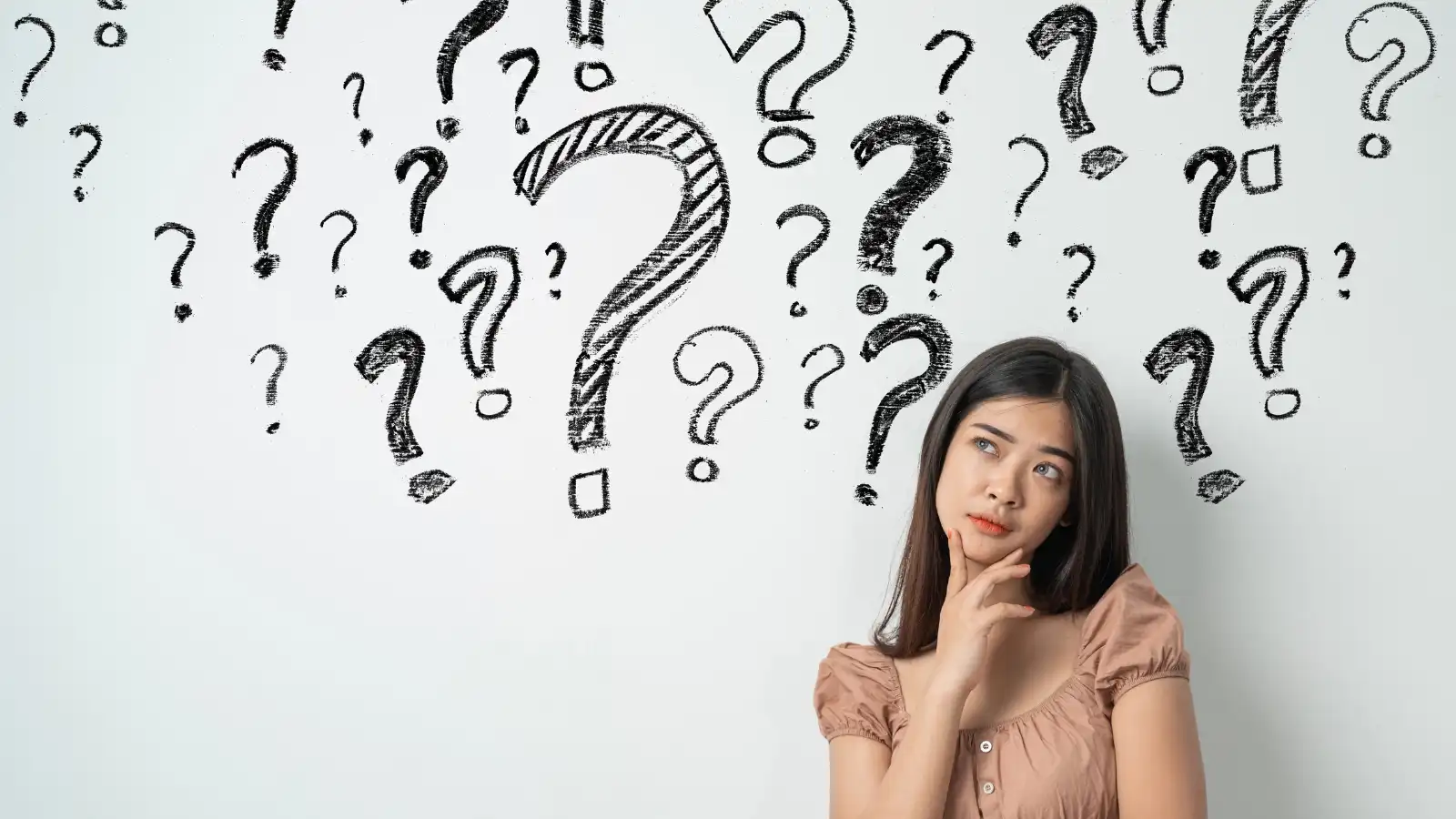
Leveraging Exogenous Data: Expanding the "Horizon" of Forecasting
Traditional demand forecasting methods focus on historical sales data, offering a limited view of potential future demand.
The retail environment is influenced by a wide array of factors, many of which are external and challenging—if not impossible—to account for using conventional models.
Weather Conditions:
Weather conditions can significantly impact consumer behavior, especially in industries like fashion or food.
For instance, a sudden cold snap may increase demand for winter clothing, while warm weather can boost sales of beverages and cooling appliances.
AI models can incorporate weather forecasts into demand prediction algorithms, adjusting inventory recommendations accordingly.Economic Indicators:
Factors such as inflation rates, unemployment figures, and consumer confidence levels play a crucial role in shaping purchasing power and behavior.
Machine learning models can continuously monitor economic trends, enabling forecasts to adapt to changing economic conditions.Social Media Trends:
Today’s consumers are heavily influenced by social media, where trends can emerge almost overnight, rapidly shifting demand.
Through natural language processing (NLP), it is possible to analyze sentiment on social media and track mentions of specific products or brands, helping retailers capitalize on trending items.Competitor Activity:
Changes in pricing, new product launches, or promotional campaigns by competitors can directly impact a retailer’s sales.
AI algorithms can monitor competitors’ activities in real time and integrate this data to refine demand forecasts.Events and Holidays:
Machine learning systems can also account for non-recurring events, such as holidays, public celebrations, or global crises, and adjust demand forecasts accordingly.This is particularly important for industries that experience spikes or dips in demand tied to specific occasions.
By leveraging exogenous data, AI can provide a more comprehensive and granular view of demand, allowing retailers to stay ahead of short-term shifts and long-term trends.
Adapting to Changing Market Conditions: A Key Advantage of AI
One of the greatest challenges of traditional demand forecasting is its reliance on static models that often fail to adapt to rapidly changing market conditions. Consumer preferences can shift suddenly due to various factors, such as economic uncertainty, political events, or global pandemics.
Machine learning (ML)-based demand forecasting systems offer a dynamic solution by continuously learning from new data and automatically adjusting predictions.
Real-Time Data Updates:
AI-powered forecasting systems are designed to process real-time data, enabling immediate adjustments based on the latest sales figures, inventory levels, or external factors.
For instance, if a retailer observes a sudden surge in online orders for a particular product, AI systems can update demand forecasts and instantly trigger replenishment processes.Behavioral Learning:
Machine learning algorithms continuously learn from changing patterns. Whether it’s a shift in customer preferences or a sudden spike in demand due to external events, AI can quickly detect these changes and adjust its forecasts accordingly.Adapting to Seasonality and Promotions:
Retailers often struggle to predict demand for seasonal products or during promotional campaigns.
AI models can handle the complexity of seasonal demand cycles more accurately than statistical models by learning from historical patterns and current market conditions.
They can also anticipate the “halo effect” of promotions, where demand increases for complementary products.
In summary, AI-driven demand forecasting not only improves accuracy but also provides agility — an essential attribute in today’s unpredictable retail environment.
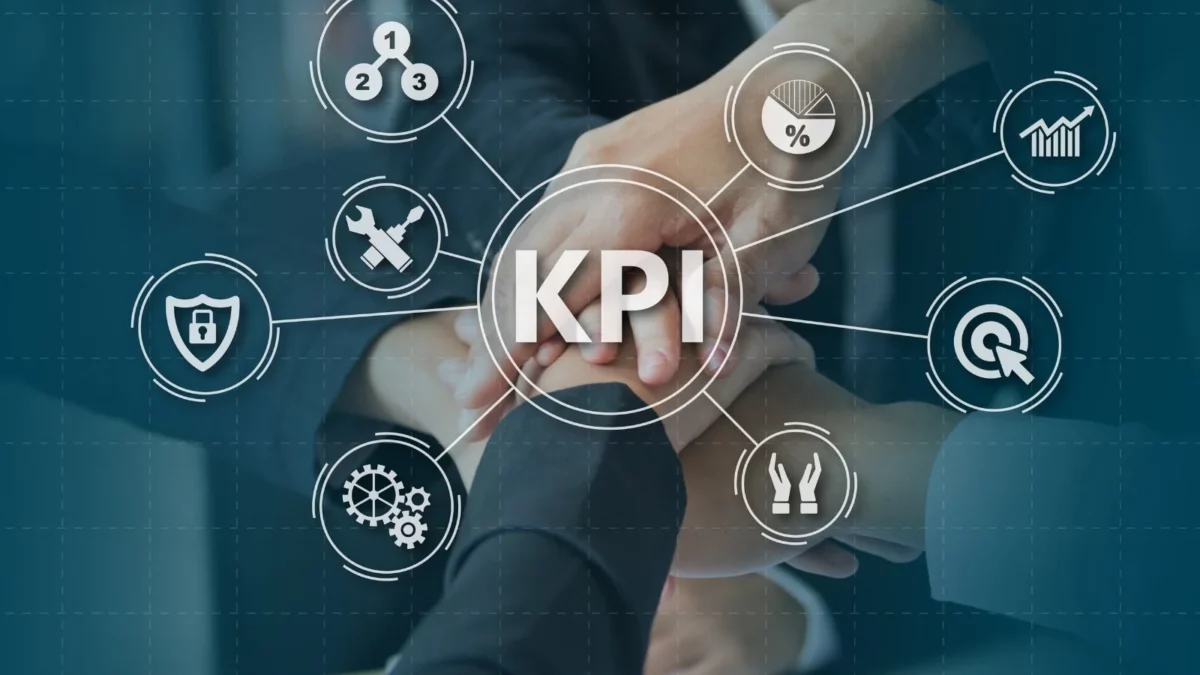
CHALLENGES AND CONSIDERATIONS FOR IMPLEMENTATION
While the benefits of ML in supply chain management are evident, businesses must remain mindful of certain challenges when adopting these technologies:
Data Quality: ML systems are only as effective as the data they are fed. Inconsistent or incomplete data can lead to inaccurate forecasts and optimization models. Establishing robust data governance practices is crucial.
Integration with Existing Systems: Many retailers still rely on legacy systems for their supply chain operations. Integrating ML solutions with these older platforms can be complex and may require substantial investments.
Change Management: As with any technological advancement, implementing AI requires organizational changes. Teams must be trained to use the new systems, and resistance may arise from those who are comfortable with existing processes.
A LOOK INTO THE FUTURE: THE ROLE OF AI IN THE FUTURE OF RETAIL SUPPLY CHAINS
As retail supply chains continue to evolve, the need to adopt more nuanced and forward-looking approaches becomes increasingly evident.
One area where ML technologies are expected to have an even greater impact is in managing seasonal shifts. Seasonality has always posed a challenge for retailers, as demand fluctuations caused by weather changes, holidays, or economic cycles require meticulous planning and precise execution.
In response to these challenges, an ML-powered seasonal strategic framework can provide the necessary tools to anticipate and prepare for these cyclical changes. By employing AI within a seasonal strategy, retailers can ensure their supply chains remain agile, cost-efficient, and capable of meeting customer expectations.
The integration of ML into retail supply chains is still in its early stages, but the potential is immense. As these technologies continue to advance, we can anticipate further innovations in areas such as autonomous delivery, advanced robotics in warehouses, and even AI-driven strategic supply chain planning.
Retailers that embrace AI will not only experience improvements in efficiency and cost savings but will also be better positioned to meet the ever-evolving expectations of customers.
In a world where disruption is the new normal, AI-powered supply chains are becoming a key differentiator in the retail sector.
THE ADVANTAGES OF A SEASONAL STRATEGIC FRAMEWORK FOR AI/ML
By integrating ML technologies into seasonal strategic planning, retailers can achieve several key benefits:
- Improved Forecast Accuracy: ML enables more precise predictions, reducing the risks of stockouts or overstocking during critical seasonal periods.
- Cost-Effectiveness: Through dynamic inventory management, retailers can lower the cost of excess stock, minimize waste, and optimize supply chain operations.
- Enhanced Customer Experience: By ensuring product availability at the right time and place, AI-driven seasonal strategies boost customer satisfaction, loyalty, and retention.
- Agility and Adaptability: In a world of increasing disruptions, AI empowers businesses to stay agile, adjusting seasonal plans in real-time to meet evolving market conditions.
The Future of Seasonal AI in Retail Supply Chains
Looking ahead, the application of AI to seasonal strategies will only become more sophisticated.
With advancements in AI-powered robotics, autonomous delivery systems, and real-time decision-making algorithms, retailers will have more control over their supply chains than ever before.
For retail supply chain professionals, adopting a strategic seasonal AI framework will be crucial to navigating the future.
By leveraging AI’s predictive capabilities, businesses can not only mitigate the risks associated with seasonality but also transform these fluctuations into opportunities for growth and customer engagement.